Gain immediate predictive insights and an actionable competitive advantage
Traditional marketing measurement approaches keep you waiting — enduring manual processes, reliant on specialized teams and receiving delayed insights while millions sit on the P&L. Change everything with Alembic. Know where you are now. Know where to position for the future.
Cost center to a revenue driver
See the true impact of your marketing in days, not months. The Alembic platform identifies which of your activities drive results to enable you to spend smarter across channels, audiences, campaigns and platforms.
Uncover hidden relationships
Discover which marketing activities influence others, Alembic uncovers relationships between campaigns that traditional analytics won’t, elevating your understanding for how brand awareness drives conversions.
Measure the unmeasurable
Alembic accurately attributes value to traditionally difficult-to-track channels including brand campaigns, sponsorships and even offline activities that make up approximately 40% of annual global advertising spend.
Get insights automatically
Alembic identifies what works, what doesn’t and where to invest next — your Intelligence Briefing provides clear concise numbers decisively proving the value of your marketing spend.
Alembic’s data operations happen at a scale so big we operate one of the world’s fastest supercomputers
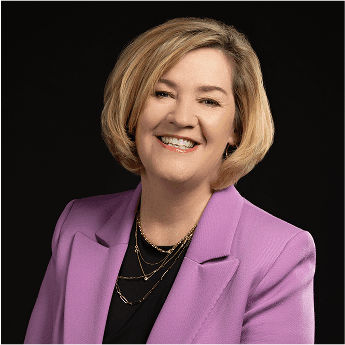
“Alembic Technologies deployed their AI infrastructure at Equinix to run proprietary inference algorithms on massive data sets for predictable cost, privacy, speed, and secure access to data sources in the cloud."
Adaire Fox-Martin
CEO & President, Equinix
FROM THE EQUINIX EARNING CALLEnterprise business impact for key industries
Alembic empowers Fortune 500 B2B technology firm to attribute marketing impact and grow sales pipeline
This Fortune 500 B2B technology leader expanded its sales pipeline by 37% using Alembics advanced causality models, enabling precise attribution and strategic growth across multiple business units.
DOWNLOAD PDF ↓Alembic transforms marketing measurement for a Fortune 500 airline
This major airline precisely measured a major sports sponsorship with Alembics advanced causality models, directly linking brand activations to ticket sales and transforming marketing from a black box into a strategic business driver.
DOWNLOAD PDF ↓Gartner® named Alembic as a Sample Vendor in their “Emerging Tech Impact Radar: Intelligent Simulation” report
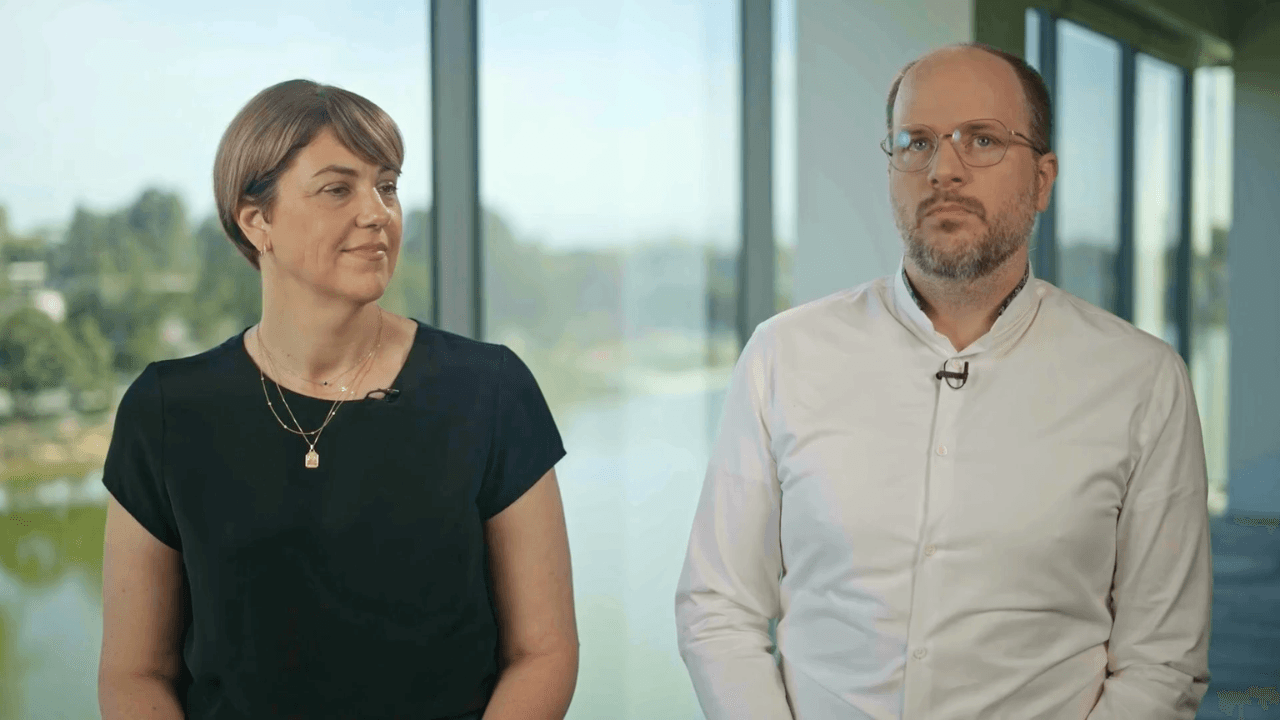
Get Alembic and go far beyond all traditional forms of marketing measurement
Extract the signal from the noise
Get clear answers from complex marketing data. Our system handles everything from data ingestion to analysis, giving you straightforward reports that make decision-making easy.
Find causality, not just correlation
Discover how marketing channels truly influence each other. See exactly how your email campaigns boost search performance or how social media impacts website conversions, with evidence, not guesswork.
Alembic offers a secure enterprise-grade solution
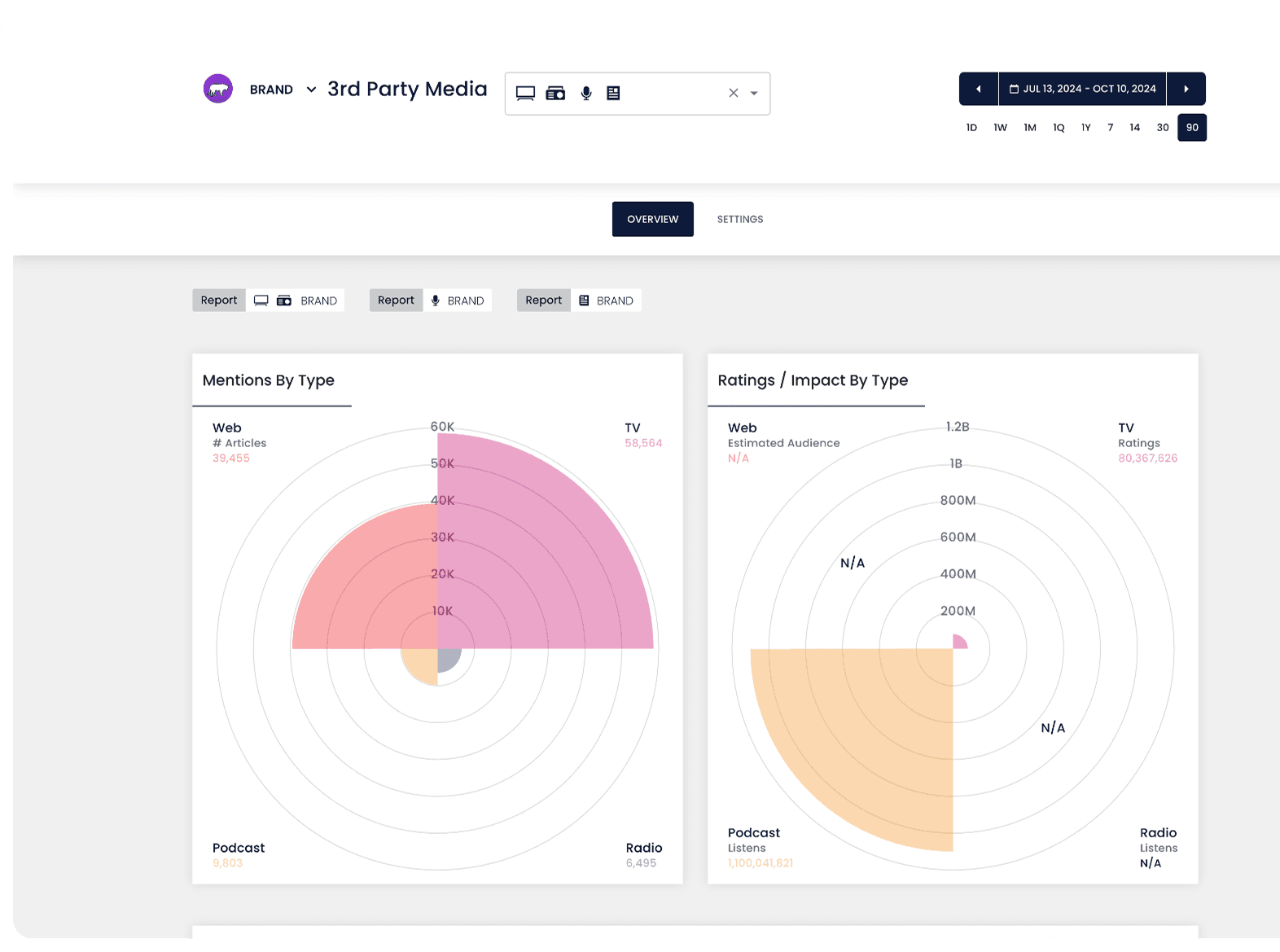
Built with enterprise in mind
We have built security into our foundation. Our platform adheres to rigorous security protocols and has achieved SOC2/3 certification, ensuring your marketing data remains protected at all times.
Privacy-first architecture by design
Our platform is built from the ground up to prevent PII collection at every stage. We use time series and aggregate data models instead of tracking individuals, ensuring complete user privacy while still delivering actionable marketing intelligence.
Your data never leaves our premises
Unlike other solutions, we keep your data fully under your control. All information remains within our secure environment, eliminating exposure risks associated with third-party data transfers.